In the current global environment where information is considered a strategic asset, organizations search for efficient systems to acquire, process, and store vast amounts of information There are two main approaches a means of storing and processing large amounts of information: data warehouses and data lakes. Organizations must consider such differences when making decisions concerning the management of the data.
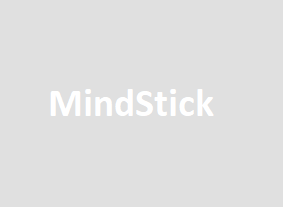
What is a Data Warehouse?
Such a data warehouse is the system that consolidates the organization’s data information coming from the sources in order to provide the structure for data analysis; Like OLTP, it is optimized to accommodate queries and analysis, rather than transactions. Which is why this process is commonly referred as Extract Transform Load or ETL and the data that is stored and arranged in a manner that is friendly to BI tools and analytical queries.
OLAP and data warehouses are designed for a fast data processing and thus they are suitable for reports, dashboards and data analysis. It affords the organization a comprehensive perspective of history and it assists in the business. Some of the popular data warehouse solutions are Amazon Redshift, Google BigQuery and snowflake.
Key Features for a Data Warehouse:
1. Structured Data Storage: Structured data is stored in the form of tables and columns which are found in data warehouses.
2. Predefined Schema: Data have to follow a certain format so that all data collected will be of good standard.
3. Optimized for Query Performance: Data warehouses are best suited for handling advanced queries and analyzing data; they have great response time.
4. Historical Data: Centered mainly on archives where data is stored for analysis of trends that would help make predictions.
5. Supports Business Intelligence: Data warehouse is an important aspect of BI and analytics, the info derived is useful for strategic purposes.
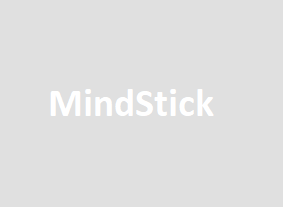
What is a Data Lake?
A data lake meanwhile is a relatively large data repository that can accommodate a substantial bulk of raw, non-ingested, or not formatted data. An important difference between data warehouses and data lakes is the fact that in data lakes there is no need to have a fixed schema for the data to be put into the data lake; data can be stored in bulk or their original format. This flexibility makes it possible that data lakes can handle not only structured or semi-structured data but it can also work with text, image, video, and sensor data and hence it is highly scalable.
Lakes of data ‘do not read the schema’ which implies that the organization of data takes place at the time of use and not at the time of deposit. This approach is useful for EDA, machine learning, and real-time analytics scenarios where several types of data are processed ad-hoc. The solutions for data lake are Amazon S3, Microsoft Azure Data Lake, Google Cloud Storage, etc.
Key Features of a Data Lake:
1. Unstructured Data Storage: Data lands can capture the data in its raw form and the structure of data land can support several types of structures and formats.
2. Schema-on-Read: A great advantage is that data is only interpreted when it is required which increases the flexibility and adaptability of an information system.
3. High Scalability: The data lakes idea is specifically developed for enlargement as the scale of the company increases to accommodate more data.
4. Supports Big Data Analytics: Best employed to solve complex problems such as data mining, big data, and real-time data processing.
5. Cost-Effective: The benefits of not transforming data include lower costs of storage as compared with data warehouses.
It is also pertinent to mention that we should be very careful while defining the differences between data warehouses and data lakes since there is a considerable overlap between the two concepts.
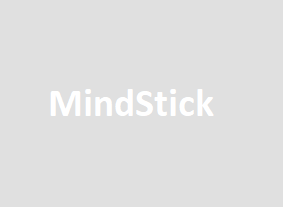
Though data warehouses and data lakes are both architectures of data storage, the basic distinction in their structures makes them useful for various tasks. Here are some of the key distinctions:
1. Data Structure:
- Data Warehouse: This is a type of database that collects data with strict organizing of how it will be done by organizing it with a fixed format or model.
- Data Lake: Stores data that do not have any specific format in a standard table structure such as column and row.
2. Data Processing:
- Data Warehouse: ETL stands for extract, transform, and load and it holds data after extracting and structuring them.
- Data Lake: Stores data in a schema-on-read approach so that it goes through rows only when it has to with a calculated process.
3. Use Cases:
- Data Warehouse: Most appropriate to be used in business intelligence, reporting, and analytics that are done on historical data.
- Data Lake: Suitable for handling large amounts of data as well as for machine learning and processing in real-time conditions.
4. Cost:
- Data Warehouse: This usually becomes costlier since data transformation, as well as data storage optimization, may be required.
- Data Lake: The system is less expensive to store bigger amounts of non-processed information.
5. Performance:
- Data Warehouse: High-performance query processor meant for structured data or relational data specifically.
- Data Lake: May need further refinement to query datasets effectively especially when working with big data.
Which One Is Better for You?
The main differences between a data warehouse and a data lake are as follows: Any organization can choose how to develop it based on its needs and objectives. But if your main goal is to quickly analyze structured data, you can opt for a data warehouse However, if you are in an environment where you have multiple types of data and would need this flexibility for Hadoop big data analytics, then Data Lake could be a better proposition.
Some organizations require all of these capabilities, and this has led to the development of a dual and more modern system. This approach combines data lakes and warehouses where raw data is stored in the pool, processed sorted, and delivered to the warehouse.
Conclusion
However, both data warehousing and data lakes have their rightful places in the modern data management framework. In analyzing the two types of ML, businesses will be in a registered position to make relevant decisions, especially when determining their data strategy and requirements. When you opt for a data warehouse data lake or even both the aim is to enable data for positive business returns.
Leave Comment