Machine learning is one of those applications of artificial intelligence whereby a computer acquires the ability to learn from data with the aim of making decisions based on it without necessarily being programmed to do so. For the last ten years, machine learning has transformed many industries, including healthcare, among others. Does machine learning improve healthcare, and how does it?
Given the overwhelming increase of populations by aging and the 'rises of chronic diseases and medical errors all over the world', machine learning has been proposed to be an innovative tool that may further enhance accuracy, efficiency, and personalized care. More specifically, we'll be diving deeper into ways in which it's already impacting healthcare and what might be its possible use for much greater change.
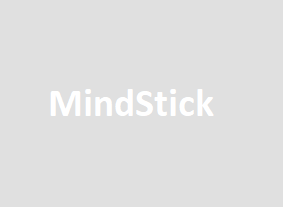
1. Machine learning in predictive analytics
Predictive analytics is one of the most visible applications of machine learning in health service delivery. Predictive analytics involves the use of previous data to predict the probable event likely to occur in the future. The processing of electronic health records, often constructed from significant datasets, allows algorithms in machine learning to predict outcomes much more accurately than traditional statistical methods. This, therefore, enables clinicians to pinpoint those who are most likely to develop diabetes, heart disease, or cancer and thus a better chance to intervene early.
For instance, patient readmission uses predictive models to identify which patients are likely to return to hospitals within 30 days of leaving the hospitals. This serves to enhance the allocation of available healthcare providers' resources and provide necessary, focused post-discharge care that may decrease readmission.
2. Machine Learning Applied to Diagnoses
Despite the sophistication of the modern delivery system in healthcare, diagnosis errors remain one of the leading challenges common to healthcare providers. . These errors have often led to wrong treatments and delayed care. Recent breakthroughs in machine learning are hugely transforming diagnostic accuracy, such as the machine learning applications used for enhanced medical imaging.
For example, in radiology, there is a collection of machine learning models that are being trained to identify abnormalities across medical images: X-rays, MRIs, CT scans, etc. These machines can recognize a wide variety of patterns invisible to the human eye and help radiologists make diagnoses and enrich their physician-service relationships.
The successful application of the use of machine learning in the detection of cancer is one of the features. At some times, AI-based tools were detected to identify cancerous tumors at an earlier age than those radiologists diagnosed. Machine learning saves lives and reduces unnecessary procedures or treatments while improving the accuracy of diagnostic precision.
3. Drug Discovery and Development
Drug development is one long, arduous, and expensive activity. Years of research and development go into putting a new drug on the market. It took years of drug research, development, and approval processes before those reached the shelves. Machine learning does much of that analysis much faster in large data sets.
ML models can predict how different compounds will react with the biological system. That is a real game-changer, speeding up drug discovery processes by far. In addition to that, machine learning even goes so far as repurposing drugs; machine learning can take approved drugs already and identify therapeutic uses for them that may not be their original approved indication.
For example, during the COVID-19 pandemic, researchers applied machine learning to search for candidates for repurposing by sifting through the relevant drug databases. It then took dramatically shorter amounts of time to discover possible treatment choices in a world health crisis.
4. Customized Treatment Plans
But another important area where machine learning impacts healthcare has to do with personalized medicine. While most treatment programs are essentially one-size-fits-all in nature, in reality, machine learning makes it possible to tailor based on genetic, clinical, and lifestyle data specifically responsive to an individual's particular situation.
For example, in oncology, machine-learning models analyze genetic data to determine the best treatment plan for every cancer patient. Predictions on how individuals will respond to therapies allow doctors to select the very best possible treatments and therefore reduce trial-and-error approaches to care.
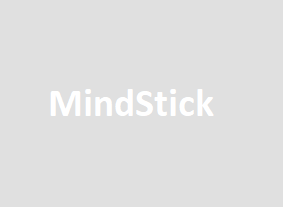
5. Operational Efficiency and Resource Allocation
It also streamlines the operational side of healthcare with machine learning. Hospitals and clinics are usually plagued by resource challenges, scheduling, and managing administrative work. Algorithms of machine learning can predict the volumes of patients, optimize staffing, and manage the supply chain accordingly in order to cut down on waste and improve efficiency.
For example, predictive models can be applied by a hospital for forewarning of the probable periods when most admissions might occur—most probably during the flu season—and hence prepare necessary medical supplies and sufficient personnel on duty. The benefits of such foresight reduce delays in patient care as well as provide resources at times of their most acute need.
6. Optimizing patient outcomes: monitoring and wearables
Wearable devices, the smartwatches or the fitness trackers, are increasingly becoming a real-time monitor for patients' health. Each algorithm type in machine learning now processes data coming from these devices as it finds the trends that may lead to increased health risks.
Such wearables will be helpful in chronic diseases such as diabetes and hypertension since they'll track the continuous system of patients' vitals. The machine learning algorithm would then process all these data streams to come up with interventions like medication adjustments or emergency visits. This thus means that patients suffering from chronic conditions that need constant monitoring will be treated sooner; therefore, their overall health outcome will improve.
7. Machine learning in telemedicine
It is a reality during the pandemic of COVID-19 to have fast growth in telemedicine; patients can receive medical care from another geographical location, and machine learning can help in this new healthcare delivery mode by giving doctors the power of predictive models that would enable them to virtually diagnose, monitor patient conditions, and make recommendations while having consultations.
In the case of triage, learning machine algorithms are even added to telemedicine. For example, how a traditional platform analyzes symptoms and medical histories with a machine learning model deciding whether a patient should come to see a physician in person or can be treated at home.
Challenges and Ethical Issues
Machine learning is promising in healthcare; however, it poses many challenges. One of them is privacy, followed by data security and biases in the algorithms. Healthcare data are very sensitive, and the consequences of breaches can be pretty sternly felt by patients.
However, machine learning algorithms are only as good as the data they are given. If biased or incomplete data are used to train such models, then the outcomes are also likely biased and will eventuate in the disparity of care.
Lastly, though machines may be so helpful to healthcare professionals, they will never replace the need for human judgment. Healthcare is a very personal and moral area, and decisions on which treatment to use involve things more complex than an algorithm can consider.
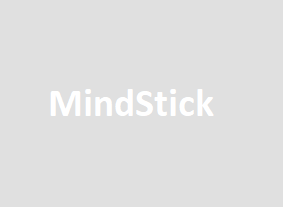
Conclusion: The Future of Machine Learning in Health Care
It transforms healthcare in so many different directions, from predictive analytics to diagnosis, from drug discovery to efficiency in healthcare operations. Yet, it is much more magnificent even in the future ahead, promising this to be the betterment of patient care, cost reduction, and saving of lives.
However, with great power comes even greater responsibility. Since machine learning is fast becoming an integral part of the healthcare system, vigilance must continue to evolve as it goes ahead to develop technology and use it in ways that ensure it is used ethically, equitably, and most importantly, always respecting patient privacy and safety.
Leave Comment