Real-time artificial intelligence embedded in edge computing is revolutionizing how industries adopt big data. Beyond the current cloud-based models, the concept of edge computing deploys AI processing at the core of data generation. This change is due to AI chips, which are dedicated processors with the function of working quickly on data in specific geographical regions. To appreciate this change, it is necessary to look at how these mechanisms enable real-time AI in edge computing. This paper looks at the application of AI chips in making real-time decisions in the edge computing environment.
1. What are AI chips, and why are they special?
AI chips are defined as those processors that are built to optimize AI-related tasks, such as ML and DL. AI chips require parallel processing, unlike the traditional CPUs or GPUs, so that different algorithms will be completed with increased speed.
Some are NVIDIA’s Blackwell chip to enhance ML training time and Google’s TPUs for efficient neural network operations (10, 14). These chips excel in:
- Reducing energy consumption.
- Raising Inference to Real-Time with Busy Latency.
- Scalability that powers high-throughput AI operations for C- and edge-level devices.
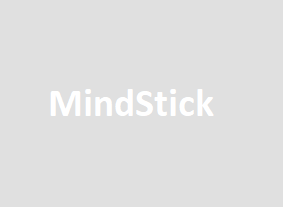
2. Character and Benefits of Edge Computing
Edge computing applies artificial intelligence closer to where the data is made—sensors, cameras, and IoT devices. This approach helps to avoid critical dependence on the centralized cloud systems and makes the decision-process faster. Edge AI chips are nowadays the core element that supports real-time AI decisions in areas such as self-driving cars, digital health, and smart cities. These chips enable:
- Reduced Latency: Through processing local data, gadgets do not have to go through a cloud server every time an action is to be made.
- Enhanced Privacy: Data is retained inside the device, thus reducing the risks of data transfer.
- Energy Efficiency: Ironically, edge devices are much more power-aware than their AI chip counterparts.
3. Advantages of the Use of the AI Chips in Edge Computing
- Reduced Latency: Processing data at the edge through AI chips eliminates delay in making decisions. For instance, chips in an autonomous car will process real-time data from its sensors in milliseconds rather than through a cloud-based system that could delay it.
- Power Efficiency: AI chips are designed for very low power consumption required in the context of IoT devices powered by batteries.
- Better Privacy and Security: With edge computing using AI chips, the need to send sensitive data over the network is removed, and this diminishes the chances of breaches.
4. Technologies That Informed Real-Time Artificial Intelligence
- AI Accelerators: These are GPUs, TPUs, and ASICs that are specialized in performing AI-related jobs. One such chip that has been developed in the past is NVIDIA’s Blackwell chip, which strongly supports training and even inference.
- Software Frameworks: Consequently, TensorFlow Lite and PyTorch Mobile are capable of facilitating developers to adapt AI models for edge deployment with the help of the chips.
Edge AI Applications:
- Healthcare: AI chips mean that monitoring patient status is real-time, and devices can identify when vital parameters are abnormal in real-time.
- Smart Cities: Real-time analysis of camera feeds in traffic management systems is enabled through the use of AI chips.
5. Pros and Cons for Appointing AI Chips at Edge
- Hardware Limitations: Faced with these problems, the design of high-performance low-power chips is difficult.
- Interoperability: Using these chips with other various edge devices can be a challenge.
- Cost: NVIDIA or Qualcomm types of chips for application in high-performance AI may be costly for implementation on large scales.
More discussion on challenges can be found in AI chip technologies.
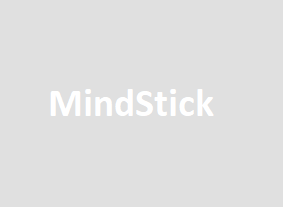
6. Variances in Industries I New Thinking and Future Trends
For edge AI applications, it has been seen that famous companies like Microsoft are developing their own unique chips for this operation. Their Azure platform incorporates such chips for real-time computations; the underlying potential remains the optimization of cost, operations, and computational performance by these technologies.
Also, future progression of 5G networks is building edge AI even more because 5G networks provide the high bandwidth and low latency needed for chip integration.
7. Conclusion
This is driving edge computing because it allows decisions to be made across the different sectors on the fly. Higher demand for faster and more localized AI computation will lead to new developments in chip technologies to make way for future real-world scenarios of intelligent systems.
The article discusses disrupting and innovating how to degrade chip technology to advance the future of AI in edge and bring real-time decision-making.
Leave Comment