Artificial Intelligence (AI) is a technology trend that has seeped into every organization and industry in the present world including medical and financial. That picture suggests that there’s more to making the most of AI than simply acquiring the technology: to get the most out of it, it’s important to know what boosts its capacity. Here are ten critical aspects that contribute to more powerful and effective AI systems:
1. Quality of Data: That is why the data are the primary and, in a certain sense, the only groundwork of any AI system. This means that the quality of the data that is fed into the AI models is high and there is a wide variety of data structures that the AI model will need to handle. Techniques such as cleaning and normalizing data are important in this process as well as feature selection.
2. Advanced Algorithms: Algorithms choice and degree of complexity determine how efficient an AI is going to be. AI system performance is defined by learning capabilities and the decision-making process that is provided by machine learning models, deep learning structures, and other AI algorithms such as neural networks or support vector machines. More research and development in the mentioned area must be conducted and maintained all the time.
3. Computing Power: Some of the AI tasks especially deep learning tasks call for a very huge amount of computation. Better processors namely the GPUs and TPUs, larger cloud computing platforms due to advanced AI algorithms help in handling huge data and enhanced models hence boosting AI.
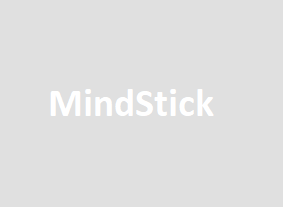
4. Data Augmentation: However, due to constraints of the number of samples, methods of data increases like rotating, scaling or even flipping are applied in this project. These methods seem to be useful in generation of more training instances, reducing errors and increasing security and efficiency of the AI models.
5. Model Optimization: Hyper parameter tuning on the existing AI models and models pruning also helps in improving the results to a greater extent. There are approaches like the grid search, random search, and Bayesian optimization for performing this action .
6. Ethical AI Practices: It can thus be clearly seen that it is important that AI systems are developed and deployed in an ethical manner for people to accept the systems and for them to be effective. Bias in AI models and lack of transparency and accountability are the major causes of the lack of trust and must therefore be addressed if AI is to reach its full potential.
7. Interdisciplinary Collaboration: Developing AI enhances when there is cross-domain cooperation, such as data science, domain knowledge, and software engineering. It makes for more creativity and implementation of real-world solutions when AI involves the expertise from both fields.
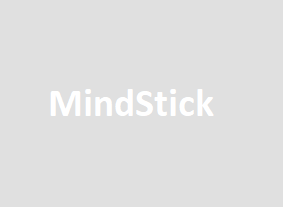
8. Continuous Learning and Adaptation: Learnable AI systems are more useful in current data and dynamic environments. Features like online learning and transfer learning let the model change its behavior over the course of its deployment.
9. Scalability: The capability of AI models to be extended or expanded will be critical when employed in the real world proc. The key enablers of scalability are the role based cloud AI services and distributed computing platforms.
10. User-Centric Design: Last but not the least, appropriate use of AI requires that the system’s design must be user centred so that the designed AI systems are not only efficient but also effective, usable and useful. This means the ability to identify users’ needs, making sure that the product is easy to use, and offering unambiguous guidelines .
Thus, IT developers and organizations can improve the characteristics and performance of the AI systems to be used in many sectors more efficiently and creatively.
Conclusion:
It will only be important to have a detailed and comprehendible grasp of these factors as AI advances further. These elements come very handy provided if you are willing to remain an innovative developer, researcher, or a business leader in the field of AI.
Leave Comment