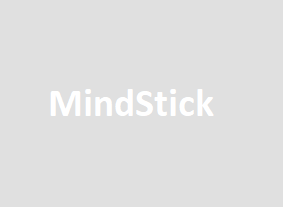
To remotely eavesdrop on phone calls, scientists employ sensors.
This important security issue was discovered by the Pennsylvania State University team using an automotive radar sensor that was readily available and a cutting-edge processing method.
According to Suryoday Basak, a doctorate candidate at Penn State, 'as technology grows more dependable and durable over time, the exploitation of such sensing technologies by adversaries becomes plausible.'
Suryoday Basak, a doctorate candidate at Penn State, stated that as technology advances and becomes more dependable and durable, it is increasingly likely that enemies will misuse such sensing technologies.
'Our proof of this form of exploitation adds to the body of literature in science that generally says, 'Hey! Audio can be intercepted with the help of automobile radars. We must take action in this regard,' stated Basak. The millimeter-wave (mmWave) spectrum, more specifically the ranges of 60 to 64 gigahertz and 77 to 81 gigahertz, is where the radar operates, which is why the researchers chose the moniker 'mmSpy' for their method. This is a portion of the radio spectrum that is used for 5G, the fifth-generation global standard for communication networks.
Researchers imitated persons chatting over smartphone earpieces for the mmSpy demonstration, which was published in 2022 IEEE Symposium on Security and Privacy (SP).
The speech causes the phone's earpiece to vibrate, and that vibration spreads throughout the phone's body. Basak explained, 'We utilise the radar to measure this vibration and recreate what the person on the other end of the connection said.
Mahanth Gowda, an assistant professor at Penn State, was one of the researchers who remarked that their method is effective even when the audio is absolutely inaudible to surrounding microphones and humans.
However, Basak noted that this particular aspect — identifying and reconstructing speech from the other end of a smartphone line — had not yet been investigated. Similar vulnerabilities or attack modalities have been discovered before.
Pre-processing is done on the radar sensor data using MATLAB and Python modules, which are interfaces for computing platform-languages used to filter out hardware-related and artefact noise. In order to classify speech and reconstruct audio, the researchers then feed this data into machine learning modules.
The processed speech is 83% accurate when the radar detects vibrations from a foot distant. They claimed that accuracy declines to 43% at six feet as the radar is moved further away from the phone.
After reconstructing the speech, the researchers can filter, improve, or categorise key terms as necessary, according to Basak. The team is still improving their strategy to comprehend how to exploit this security weakness as well as how to better defend against it.
The approach we created can also be used to detect vibrations in smart home systems, building monitoring systems, and industrial machinery, according to Basak.
The researchers claim that similar systems for home upkeep or even health monitoring may profit from such sensitive tracking.
Imagine a radar that could follow a person and alert authorities if any worrisome changes are detected in any of their health parameters, suggested Basak. Radars in smart homes and businesses can facilitate a quicker turnaround when difficulties and concerns are recognised, the author continued.