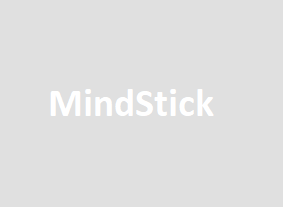
OpenAI Explores New Paths as Current AI Methods Hit Limits
OpenAI has the ambition to look beyond that because they hit the wall of what is possible with current-day methods when creating their AI tools, such as the very capable ChatGPT and other AI gadgets. AP: Ninety-nine percent of models trained today, nevertheless, are far from perfect. Today’s AI models are already quite good, but it seems that they’re getting to the point where traditional ways of adding more capacity to the model or feeding more data into it are becoming ineffective.
Therefore the questions arise: what is the next step for OpenAI, and why are all these new modifications necessary? Why AIbib's Current AI Approaches Aren’t Enough
Contemporary AI and the giant language models on which OpenAI’s ChatGPT is based depend on equally enormous data volumes and computational resources. This approach, known as'scaling’, is fundamentally at the root of a great deal of AI’s recent advances. By feeding these models more data and increasing the size of what developers call the model, they have trained artificial intelligence that can write human-like text and recognise images.
However, this comes with issues when scaled, This means that as things are scaled, some factors arise. First, it’s costly: training these models requires significant amounts of energy as well as capital. Second, scaling does not address the core issues of intelligence—reasoning, understanding context, and most real-world decision-making—which continues to elude AI. OpenAI is aware of these problems and understands that if AI is to progress, it is going to have to do things differently.
To overcome these limitations, OpenAI is looking for more sustainable and progressive AI methodologies. That’s precisely what they are said to be funding—their research priorities include algorithmic improvement, a direction that extends beyond just the size of the models but to its overall efficiency. Another is reinforcement learning and symbolic AI, both of which could help make AI more efficient at reasoning and learning in a more bureaucratic way.
Further, OpenAI has also teased the idea of improving AI’s capacity to learn in self-supervised ways with limited guidance, which should translate to models that are better able to generalise to new tasks without needing extensive retraining. These new directions are to create AI systems that are comparatively not only eager for data but are also able to read and respond to situations differently.
It must be clear to anyone who is at least familiar with the current state of AI what this means for the future of AI.
Should the new directions be pursued successfully, then they can genuinely revolutionise the development and application of AI. For users, this could mean flexible AI systems that better serve user needs and require less direct ‘AI intervention’.
Though these changes will not occur in the near future, OpenAI’s devotion to further advancements implies that AI’s role in our lives will become even more tightly woven in, a process that will not be hindered by modern technological capabilities.