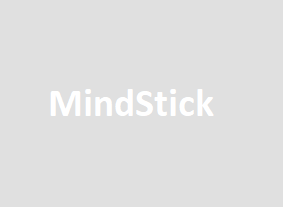
What if algorithms get innovative
In our body-brain is the main functioning part. The brain is highly adaptable. Every day, we create new memories, learn new things, and improve our talents. This is in sharp contrast to today's computers, which normally only carry out pre-programmed tasks. Synaptic plasticity is at the heart of human flexibility. Synapses are the places of connection between neurons that can alter depending on how they are used. Synaptic plasticity is a hot topic in neuroscience right now because it's crucial to learning and memory. Experts in the domains of neuroscience and artificial intelligence (AI) are developing models for the principles underlying these brain processes to better understand them and design adaptable robots. Such learning and plasticity models aid in the understanding of biological information processing and should also allow machines to learn more quickly.
Algorithms that the mimic biological evolution :
Researchers at the University of Bern's Institute of Physiology have created a new technique based on so-called evolutionary algorithms while working on the European Human Brain Project. These computer programs replicate the process of biological evolution, such as the concept of natural selection, to find answers to issues. As a result, biological fitness, which refers to how well an organism adapts to its surroundings, serves as a paradigm for evolutionary algorithms. The 'fitness' of a candidate solution in such algorithms is determined by how well it solves the underlying problem.
Fantastic innovations :
The 'evolving-to-learn' (E2L) or 'becoming adaptive' approach is the name given to the newly created method. The research team, coordinated by Dr. Mihai Petrovici of the University of Bern's Institute of Physiology and the University of Heidelberg's Kirchhoff Institute for Physics, put evolutionary algorithms to the test in three different learning scenarios. The computer had to recognize a repeating pattern in a continuous stream of information in the first case without receiving any feedback on its performance. In the second scenario, the computer was rewarded with virtual benefits for acting in a certain way. Finally, in the third 'directed learning' case, the system was told just how much its behavior differed from the anticipated one.
'The evolutionary algorithms were able to find mechanisms of synaptic plasticity in all of these cases, and so successfully addressed a new job,' explains Dr. Jakob Jordan, corresponding and co-first author from the University of Bern's Institute of Physiology. In doing so, the algorithms demonstrated incredible ingenuity: The algorithm, for example, discovered a new plasticity model in which the signals we defined are joined to create a new signal. We see that networks employing this new signal learn quicker than networks using previously known rules,' says co-first author Dr. Maximilian Schmidt of the RIKEN Center for Brain Science in Tokyo. The findings were published in the eLife journal.
'We consider E2L as a potential strategy for gaining deep insights into biological learning principles and speeding up progress toward powerful artificial learning machines,' Mihai Petrovoci explains. 'We think it will hasten research into synaptic plasticity in the neurological system,' Jakob Jordan concludes. The discoveries will provide light on how healthy and sick brains function. They could also pave the way for the creation of intelligent machines that can better respond to their customers' requirements.
Read More: https://www.mindstick.com/news/797/ai-to-design-the-next-gen-artificial-intelligence-chip-faster-than-humans-google